The growing complexity of pricing models coupled with an ever-evolving regulatory landscape means that it has become imperative for insurers to implement AI governance – in other words, the controls that ensure AI is developed and deployed in a transparent, ethical, efficient and trustworthy way. Selim Cavanagh writes
However, scaling AI governance across large businesses that may deploy hundreds of different models is not an easy feat, especially when they are managed manually in-house.
Why is scaling AI Governance a challenge?
On July 31st, the Consumer Duty will come into effect in the UK, which will maintain that insurers must offer fair value and outcomes to their customers. Failure to comply with these new rules will result in customer dissatisfaction, reputational damage, and staggering fines.
Governing a single model to operate within these parameters whilst still delivering value to the business is one thing, but large insurers might have hundreds of AI models in production across their business. The majority of these are likely to be in their pricing department, where multiple models work together in complex ways to reach an optimal price quote. Each pricing model requires specific data, performs a specific function, and as a result of the Consumer Duty, each model will soon require necessary levels of explainability for its outputs to be examined and understood by various stakeholders.
If you are an insurer looking to scale your AI capabilities, governance is something that you need to master. Here are seven steps that can make this a success:
1. Create transparent KPIs and boundaries for model monitoring
How well do you really know your competitors?
Access the most comprehensive Company Profiles on the market, powered by GlobalData. Save hours of research. Gain competitive edge.
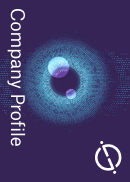
Thank you!
Your download email will arrive shortly
Not ready to buy yet? Download a free sample
We are confident about the unique quality of our Company Profiles. However, we want you to make the most beneficial decision for your business, so we offer a free sample that you can download by submitting the below form
By GlobalDataYou will need to understand what “good” looks like from a monitoring standpoint, so you can identify when and where a model isn’t performing as it should. This could be for various reasons including data drift, which affects nearly all machine learning models within their first year, and can have a negative impact on model performance if not addressed.
To effectively deal with data drift, insurers can implement protocols for regularly monitoring and updating their models, so they can ensure AI models can scale and deliver ROI post-deployment.
2. Conduct comprehensive risk assessments
The Consumer Duty also means that insurers will be obliged to demonstrate that their pricing represents fair value to their customers by sharing clear, understandable explanations for why their insurance premiums are priced a certain way, ensuring transparency and building trust. Demonstrating a customer-centric approach will reduce the likelihood of customer complaints, which will indicate to the FCA that your pricing models are operating within the parameters of the regulatory framework. In most mature insurance markets there is a clear exponential trend towards a high volume of complex Machine Learning models deployed within pricing which is complicating explainability.
To solidify these efforts and ensure necessary transparency standards are met, insurers can do things like documenting the criteria and rationale underlying these pricing outcomes.
Explainability to other stakeholders is equally as important, for example business professionals, the regulators themselves, and technical professionals. All of these examples need to be explained in a different way. Technical professionals, like Data Scientists, may look at specific algorithms and the techniques that make the complex decision-making processes of AI models transparent.
This can include methods to visualise the feature importance in predictive models or techniques like SHAP (SHapley Additive exPlanations) to explain the output of machine learning models. Less technical business professionals, on the other hand, will naturally focus more on the governance and policy implications – how it impacts customer relations, meets legal standards, and integrates with business strategies.
4. Quantify model performance
Once the necessary levels of scrutiny have been established, insurers will need to define the operational bounds for their AI systems to ensure reliable model performance. Setting benchmarks for model consistency and incorporating feedback loops will provide a foundation for your model’s performance, upon which you can build so your AI systems improve over time.
5. Anticipate and be ready for the market’s changing dynamics
As pricing solutions are a dynamic area of the market, they require constant improvement. If insurers create a culture of data-driven decision-making and adaptability within their pricing teams, it will enable them to react quicker to changes within the market and stay ahead of the competition. Utilising models, technologies and AI systems that continuously learn and have been purpose-built to auto retrain and adapt to changes they see in data will allow insurers to stay ahead of the curve, without any additional investment in headcount.
6. Define clear lines of accountability for risk ownership
AI governance necessitates the involvement of multiple stakeholders, and is vital in a core business function like pricing, so all employees understand their level of accountability for risk within their respective areas. This includes maintaining compliance with regulatory mandates, such as the EU AI Act and ISO/IEC 42001 standards.
7. Don’t do it alone
It would be impossible for an organisation to possess all the necessary resources, technology, and skills they’d need to adopt AI and maximise its full potential. Establishing partnerships with AI experts in the insurance space can give insight into best practices for risk management and regulatory compliance.
The benefits extend beyond compliance
Some insurers have already realised the importance of AI governance and have spent small fortunes on the people and hours required to do all this manually. The process of implementing it has probably resulted in creating an appreciation for these issues, and an organisation-wide culture of AI safety which will be valuable in the years to come.
3On the other hand, the same process, because of how time-consuming and challenging it is, has potentially created a sense of diminishing returns and a feeling that the true value of AI is not being realised.
Luckily, just as the rules affecting how every organisation builds, deploys, and uses AI are changing, new solutions for adapting to these changes, automating management and delivering superior outcomes are emerging. AI Governance is no longer something to talk about. It’s a reality, and the time for action has arrived.
Selim Cavanagh is the director of Insurance at Mind Foundry