For over a decade, general insurers have used price optimisation techniques to provide insight, yet the use of these tools is still limited in the life market. Alastair Black, a senior consultant with Willis Towers Watson and Alan Clarkson, a senior pricing actuary with The Royal London Group explore how they techniques are starting to be used in the life insurance industry. .
Insurers would generally like to know what value customers place on their brand, product quality and service levels.
They may wish to flex prices in order to increase volumes such that they match maximum servicing capacity.
Alternatively, they may want insight into how much weight advisers put on competitive quotes, and how close to the top quote in the market pricing needs to be in order to win business.
For over a decade, general insurers have used price optimisation techniques to provide insight into these issues, yet the use of these techniques is still limited in the life market.
Therefore, there exists an opportunity for insurers to develop more sophisticated and granular pricing approaches that can be used to better understand their business and to increase profit margins and/or improve volumes.
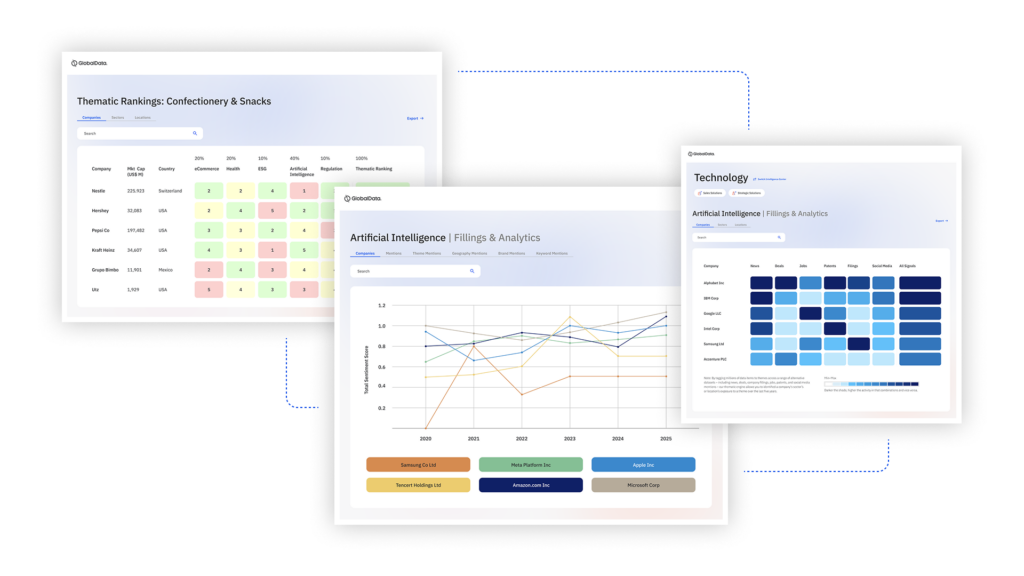
US Tariffs are shifting - will you react or anticipate?
Don’t let policy changes catch you off guard. Stay proactive with real-time data and expert analysis.
By GlobalDataWhat is price optimisation?
Price optimisation separates the ‘risk’ component of pricing from the ‘demand and elasticity’ component.
The risk part is the cost of providing the policy. Life insurers recognise the importance of accurately understanding the risk component and are generally good at this.
The demand and elasticity part explores the propensity of potential customers to purchase a product, as a function of customer characteristics and by the price charged.
Examples that may influence demand (over and above the insurer’s price relative to its competitors) are age, sum assured, socio-demographic status, policy options selected and distribution channel.
Demand and elasticity is commonly modelled using generalised linear models (GLMs). These allow for the different factors that influence demand and the interactions between them. This modelling requires the analysis of historical quote data for both customers who go on to buy and those who don’t – the latter being a rich but neglected source of information.
An insurer’s pricing team can gain a granular understanding of both (A) the profit per policy sold (from risk component modelling) and (B) how the probability of purchase (from demand and elasticity component) varies as the premium changes. These components can then be combined to produce (C) the expected profit for different premiums (Figure 1).
Balancing profit and volume does not need to be done at a high level – it can be completed at each granular level, helping to determine an overall set of prices that optimises the insurers’ capital and sales opportunities.
For example, in Figure 1 the price selected is not the one that maximises profit, but a lower price that may meet higher volume targets, or improve customer value.
Price optimisation modelling allows the impact of varying pricing strategies to be predicted using detailed evidence-based analysis rather than mainly relying on judgement and experience.
Considerations in implementing Price Optimisation techniques
Current approaches often mean that companies don’t explicitly ask (or answer) questions around customer fairness, potentially leading to cross subsidies within the existing pricing regime.
Price optimisation modelling provides an understanding of customer behaviour at a granular level, so it is important that insurers set out customer fairness principles.
Considerations would include:
- What is the maximum profit margin we will make on any one customer?
- What is the maximum difference in price we will charge two customers with similar underlying risks?
- What is the maximum level of cross subsidies between customers we will accept?
Practical considerations around data sources are also important. For example, a conversion rate of “quotes to new business” could be used as the demand measure.
However, a significant volume of quotes in the protection market offer no direct sales opportunity – they are produced by advisers and providers checking prices. It is important to isolate real quotes in order to build up a true picture of current demand and how this may be impacted by a change in price.
Why have these techniques been used less in life insurance?
In general insurance, the majority of business is written directly to the customer. Customers provide lots of details upfront and a firm price is provided.
The prices vary considerably for different customers and the presence of aggregators and use of external data sources mean that a motor insurer generally has hundreds of rating factors available for its analyses.
In life insurance the majority of business is sold through financial advisers with business typically sold on guaranteed premiums with terms of 20-30 years.
Providers quote ‘standard prices’ based on a low numbers of rating factors – typically sum assured, age, term and smoker status.
A firm (underwritten) price is not provided until a full application with medical questions has been completed. Some customers will then be offered a higher price to reflect their higher risk, but most customers will continue to be offered the ‘standard price’.
This lower availability of data means that it is more difficult to implement as rich a price optimisation model in life insurance. There are, however, differences in this market that whilst presenting challenges also offer opportunities:
- As well as customer related factors, adviser related factors, including the level and shape of commission, how this fits with an advisers’ distribution model and the price sensitivity of an adviser, can be a significant influence on demand and elasticity.
- A standard price is initially quoted followed by an underwritten price.The decision made by customers when the price does or doesn’t change is potentially a valuable source of information.
- There is a movement within advised business to request more information to provide an initial quote.This information, along with advanced data analytics techniques, offers a life insurer a greater insight into their potential customers.
- The use of price comparison sites is a small part of the life insurance market but their use is growing.This is likely to make customers more price sensitive, and so this channel will become more of a focus for price optimisation techniques in the future,
What is the business impact?
Price optimisation techniques give insurers a better understanding of their customers which, alongside the development of a robust customer fairness framework, should drive improved business outcomes.
In a competitive marketplace, being able to predict the impact of price points based on a detailed understanding of your customers could be a big opportunity for life insurers, allowing them to more effectively manage capacity and balance this with demand. It could also help identify areas of the market currently not ‘converting’.
For example, conversion rates may be poor for smaller sum assureds, which could be improved by offering a lower but still profitable price. Ultimately, the use of these techniques allows profitability, volume and customer value objectives to be met using fact rather than judgement.